Opened special issues
The last special issue (SI) published in the Radioengineering Journal dates back to the year 2015. This SI was focused on the emerging topic of memristors and their applications. The papers published within this SI were authored by leading experts in the field, such as Prof. Chua, Prof. Biolek, Prof. Tetzlaff, and many others. These papers have already received numerous citations, making the SI highly successful.
Now, after eight years, the editorial staff of the Radioengineering Journal has decided to open a new limited number of SIs. The topics for these SIs will be carefully selected with respect to scientific soundness, application potential, and impact on the engineering community.
The journal's editorial staff offers a very fast review process, with a maximum duration of two weeks to reach a first-round decision. This process is led by distinguished experts who specialize in the research areas of the respective SI. Additionally, papers accepted for any SI will receive a 20% discount, reducing the final publication fee to 320 EUR. To apply this discount, authors of accepted papers must contact chief editor chief@radioeng.cz before generation of invoice. It is important to note that SI does not represent a closed journal volume or number, as accepted papers will still be published on-the-fly.
Please read important note here. To start regular review process, all papers must be uploaded via online submission system of the Radioengineering journal. The authors of papers must use the recommended journal template in MS Word Template DOC or Tex Template TEX. Papers prepared in another journal template will be automatically rejected!
4: Power System Modeling Based on Multi-Objective Optimization and Intelligent Algorithms
In recent years, algorithms have a great achievement in the single objective optimization task, but the optimization problem in power system modeling is complicated and non-differentiable, which is difficult to be solved out with traditional mathematical methods. Moreover, it’s not enough to solve the problem in the actual power system operation. Hence the emergence of intelligent optimization algorithms such as genetic algorithm, particle swarm optimization algorithm, ant colony algorithm and neural network algorithm has shown strong and effective ability in multi-objective optimization problems of power systems. Therefore, the important research direction for power system modeling application is how to introduce muti-objective optimization problem into algorithms.
This special issue aims to provide a platform that allows active researchers to present their researches and review articles that concern of power system and algorithm. The special issue’s research area with a strong focus on power system optimization modeling, intelligent algorithm, multi-objective optimization, as well as smart grid. Topics that are invited for submission include (but are not limited to):
• Hybrid model of multi-objective optimization algorithm in power system;
• Machine learning with power system optimization modeling;
• Power flow calculation and optimization problem of power system;
• Reactive power optimization objective and power flow optimization for power system operation;
• Energy storage optimization of distribution network on multi-objective particle swarm optimization;
• Multi-objective reactive power optimization algorithm for system network loss;
• Multi-objective optimization of wind power system modeling with hybrid intelligent algorithm;
• Multi-objective optimization operation strategy of battery energy storage system;
• Genetic algorithm in multi-objective power grid optimization.
Prospective contributors are asked to write a note in cover letter that their paper belongs to the SI: Power System Modeling during the initial paper upload process. Deadline for initial paper submission is 1st March 2025. Here, you can find, download and distribute document Call for Papers.
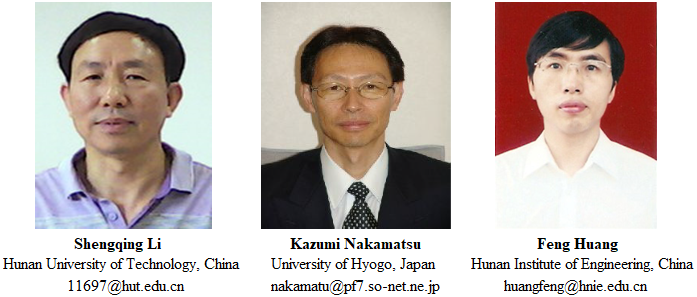
5: Signal Processing Techniques for Improved Underwater Communications in Harsh Environments
In recent years, there have been significant developments in signal processing techniques to improve underwater communications in harsh environments. Underwater communications in harsh environments can be challenging due to various factors such as attenuation, multipath propagation, and interference caused by the underwater environment. However, signal processing techniques can be used to improve underwater communications in these conditions. One of the techniques that can be applied is adaptive equalization, which reduces intersymbol interference caused by multipath propagation. Additionally, channel coding techniques can be used to improve the reliability of underwater communication systems. This is achieved by using error-correcting codes to detect and correct errors introduced by the channel. On the other hand, Orthogonal frequency division multiplexing (OFDM) is another technique that can be employed to combat multipath propagation. By spreading the data across multiple subcarriers, the data is more robust against interference and attenuation. MIMO techniques, which utilize multiple antennas at the transmitter and receiver, can also improve the quality of the received signal. This approach mitigates the effects of multipath propagation and improves the signal-to-noise ratio. Furthermore, time-reversal is a technique that can be used to focus a transmitted signal in a specific direction. Underwater acoustic positioning techniques can also be used to locate underwater objects or vehicles using the time difference of arrival (TDOA) or the phase difference of arrival (PDOA) between signals received by multiple sensors. These signal processing techniques can help mitigate the effects of multipath propagation, interference, and attenuation caused by the underwater environment, thereby improving the reliability and quality of underwater communication systems.
This special issue aims to explore and present recent advances and developments in signal processing techniques for enhancing the reliability and quality of underwater communication systems. The issue seeks to bring together research and practical applications of signal processing techniques in addressing the challenges posed by the underwater environment, such as attenuation, multipath propagation, and interference. Topics that are invited for submission include (but are not limited to):
• Investigating the effectiveness of beamforming techniques for underwater communication in highly turbulent environments;
• Developing adaptive equalization methods to mitigate the effects of multipath propagation in underwater acoustic channels;
• Examining the use of MIMO systems for enhancing data rate and reliability in underwater communications;
• Impact of oceanographic conditions on the performance of underwater communication systems;
• Robust coding schemes for efficient transmission of data over long-range underwater channels;
• Analyzing the use of underwater acoustic networks for monitoring and surveillance applications in harsh environments;
• Designing novel signal processing algorithms for improving the accuracy and resolution of underwater imaging systems;
• Acoustic fingerprinting techniques for underwater localization and tracking of objects and animals;
• Feasibility of using machine learning for adaptive signal processing in underwater communications;
• Ambient noise on the performance of underwater communication systems and developing noise reduction methods;
• Innovative underwater communication protocols to ensure efficient and reliable transmission of data in harsh environments;
• Multi-carrier modulation techniques for achieving high data rates in underwater communication systems.
Prospective contributors are asked to write a note in cover letter that their paper belongs to the SI: Signal Processing Techniques during the initial paper upload process. Deadline for initial paper submission is 25th March 2025. Here, you can find, download and distribute document Call for Papers.
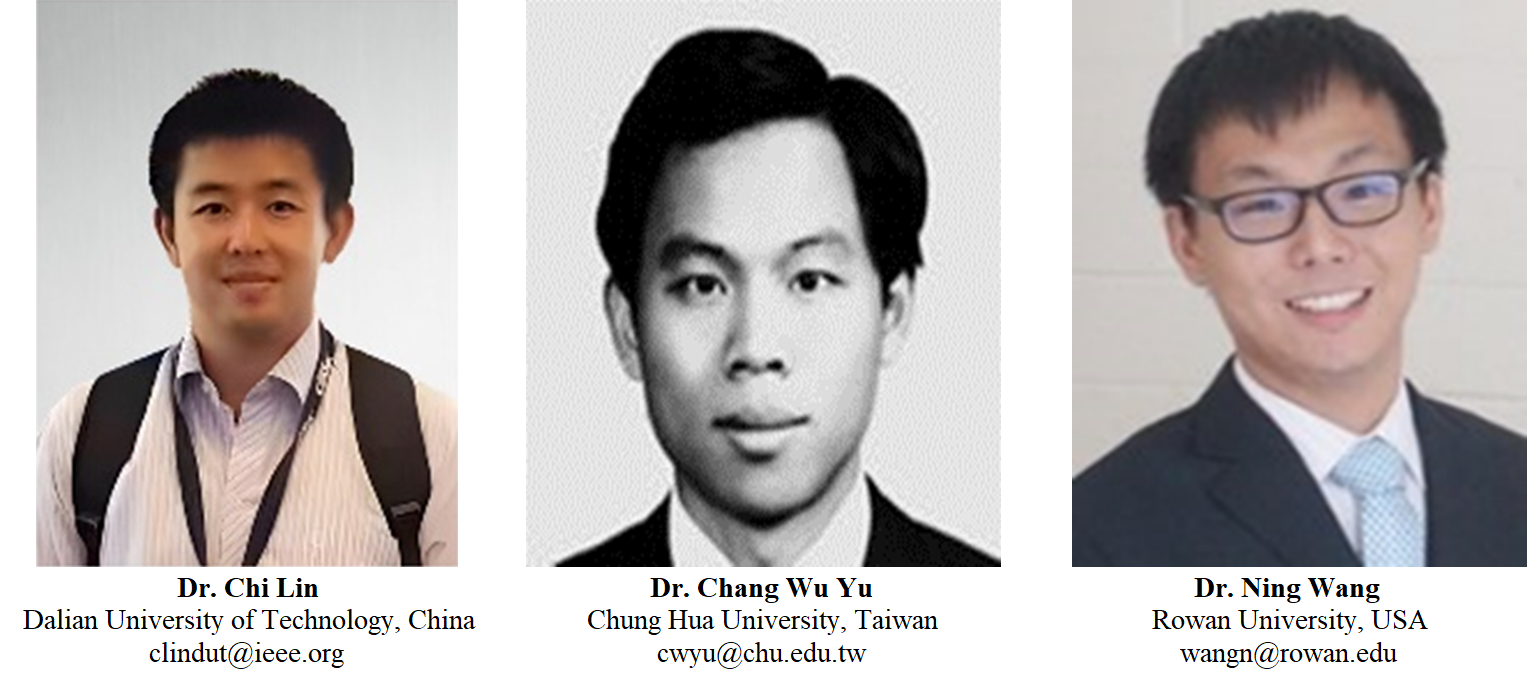